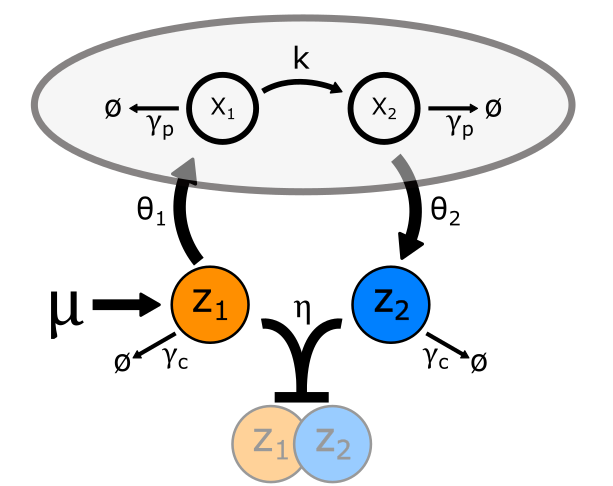
Homeostasis is one
of the central processes that pervades all of life. Each organism must regulate its
internal state, be it a warm-blooded vertebrate regulating its temperature or a
single bacterium balancing its osmotic pressure. To do this, they generally rely on
feedback control. While it has long been known that feedback control exists in
biology all the way down to the molecular level, it has so far been difficult to
engineer reliable feedback regulation into cells to perform synthetic functions.
This has changed in recent years, with the development of a simple mechanism for
implementing precise adaption in cells known as Antithetic Integral Feedback (AIF).
This mechanism has the fortunate properties of both being simple to design using
known biological parts and having certain theoretical guarantees of its performance.
Our work uses tools from control theory to describe a set of mathematical
relationships that impose strict performance tradeoffs and hard limits on such a
circuits behavior. These can be thought of a system of guidelines that can help to
inform the design of synthetic biological feedback systems.
This work was published in two papers, one in Cell Systems and one in
iScience. The work was done in collaboration with Ania Baetica, Fangzhou
Xiao, and Yoke Peng Leong, with advisement from John Doyle and Richard Murray at
Caltech.